Artificial intelligence methods and tools
Artificial intelligence (AI) methods and tools are fundamental to developing and deploying effective AI solutions across various fields. Understanding these methods enables practitioners to create more accurate models, streamline processes, and solve complex problems with greater efficiency. By leveraging the right tools, AI practitioners can enhance the performance and scalability of their systems, making AI more accessible and powerful.
Machine Learning (ML) Techniques
Essential for building predictive models, ML techniques like supervised, unsupervised, and reinforcement learning allow for diverse applications in data analysis and decision-making.
Deep Learning
A subset of ML, deep learning focuses on neural networks that can automatically learn features from large amounts of data, leading to breakthroughs in image recognition, natural language processing, and more.
Natural Language Processing (NLP)
NLP tools enable computers to understand, interpret, and generate human language, playing a crucial role in applications like chatbots, translation services, and sentiment analysis.
AI Development Tools
Environments like Jupyter Notebooks and frameworks like TensorFlow and PyTorch are critical for developing, testing, and deploying AI models, providing the infrastructure needed for modern AI projects.
Methods and Tools in AI
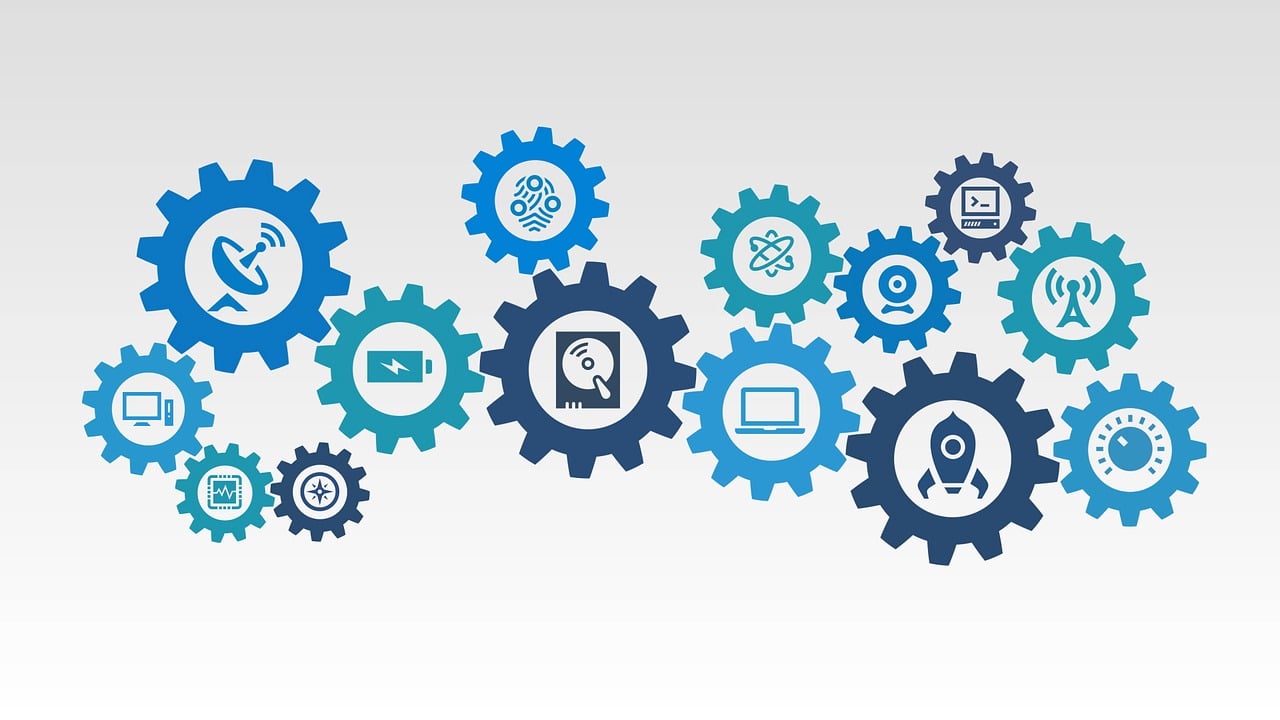